Tired Of Manually Reviewing Disparate Data? Try Integrated Data Review
By Indrani Kakade, consultant
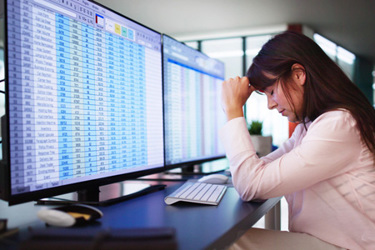
Today’s clinical data streams are complex, heterogeneous, and often incompatible, challenging the most seasoned clinical trial team members. While EDC systems are still the primary source of data collection, the adoption of DCTs has led to a surge in patient-generated data from sources like eCOA, ePROs, wearables, and implants. Additionally, real-world data sources such as EMRs and EHRs are increasingly used to facilitate patient recruitment, identify eligible study populations, and analyze large datasets. However, clinical trial data cleaning methods have been fragmented, siloed, and primarily a batch-wise, sequential process relying on a combination of manual reviews and automated checks, resulting in cumbersome reviews. These traditional data management methods are no longer sustainable, so companies are adopting a more integrated, proactive, and technology-driven approach for real-time data review and analysis: The integrated data review (IDR).
Recently, IDR has emerged to offer a unified, real-time approach to managing clinical trial data by consolidating diverse datasets on a single platform. IDR represents a fundamental shift in how sponsor companies and their partners manage clinical trial data, moving from a data-driven to a data-optimized process. The platforms that drive integrated data reviews can conduct multi-source and seamless data ingestion, integration, processing, and analysis. This evolution is enabling a panoramic view of data to help research teams ask better questions that lead to deeper insights and more efficient trial execution.
The Evolving Role Of IDR In Clinical Data Management
An IDR system consolidates data from multiple sources — EDC, vendor databases, and real-world evidence — providing a holistic view of study health. With real-time access, study teams can accelerate data cleaning, resolve issues efficiently, and streamline trial management. These platforms leverage persona-based automated data reviews and real-time analytics, facilitating faster decision-making. Shared workspaces and collaborative tools further enhance efficiency, improving cycle times and final data quality while ensuring compliance with regulatory standards such as 21 CFR Part 11.
One of the biggest advantages of IDR is its ability to streamline and automate data review processes. Traditional data reviews rely heavily on manual reviews, edit checks, reconciliation across multiple databases, and data sources. Manual reviews are performed by data managers, CRAs, and medical monitors and involve checking data across multiple sources to ensure complete and accurate collection and reporting. Depending on trial complexity, manual reviews can take weeks or even months with each review cycle that involves multiple back-and-forth communication between stakeholders, leading to potential delays in data cleaning and analysis.
With IDR, automated checks and algorithms can detect inconsistencies and highlight potential issues early in the trial, allowing study teams to address them promptly. For example, the early detection of adverse events (AEs) through real-time monitoring of PROs, labs, concomitant medications, imaging data, etc.
What To Look For In IDR Functionality
There are multiple platforms in the market offering IDR functionality. Most systems provide collaborative workflows and shared spaces for real-time data reviews by clinical teams, data managers, statisticians, programmers, and others. This ensures improved oversight and communication along with reduced risk of misinterpretation and redundancies. For example, the safety reviewer may notice an increase in a particular adverse event, and this is flagged for a review by the statistician and clinical team. This may lead to causality assessment and the need for a sub-group analysis or protocol amendment.
These platforms are now leveraging AI for automated data ingestion, validation, mapping, advanced analytics, and visualization. Teams are using these features to identify and predict potential risk (adverse events, patient dropouts, missed recruitment, etc.) and suggest mitigation strategies, further improving trial outcome. Sponsors are using patient data to track compliance and potential dropout risks. For example, in an oncology Phase 3 study, the compliance or adverse pattern can be tracked to predict a potential dropout for specific patients. This can be mitigated through proactive engagement steps and targeted interventions like follow-up phone calls, reminders, or transportation support to improve retention.
Lastly, the IDR platforms are designed in compliance with regulatory guidelines and standards such as ICH E6(R3) and data security requirements such as 21 CFR part 11, GDPR, and HIPAA.
How To Address Challenges In IDR Implementation
Despite its numerous advantages, implementing IDR presents several challenges that must be proactively addressed. The initial infrastructure cost of implementing IDR is high, depending on various factors that include the level of complexity, customized features, commercial vs custom-built offering. These costs can vary between $ 200,000 to $1.5 million for a custom development plus ongoing maintenance. Data security and privacy are also critical concerns, necessitating robust security measures and data transfer agreements between vendors. Integration complexity and system interoperability can be a challenge that requires data mapping and standardization. These platforms must be built to scale up to ingest multisource and large datasets.
Lastly, it is important to consider the change management aspect during implementation of an IDR platform. The teams accustomed to traditional data review processes may resist changes in process and technology adoption. Proper training on revised SOPs, clear communication, and phased rollouts are essential to ensuring a smooth transition.
The Future Of IDR In Clinical Trials
IDR is reshaping data review from a reactive to a proactive process. AI-based automation further strengthens this process, enabling faster decision-making, regulatory compliance, and efficient trial execution.
As the industry progresses, IDR adoption will continue to evolve, driven by technological advancements and regulatory guidelines. Newer features like agentic AI — those with decision-making capabilities — are being explored for automated data query management. Companies are also using the approach of hyperautomation to enhance efficiency by automating repetitive and operational tasks like data ingestion, processing, quality checks, thus reducing manual effort and improving data quality. By integrating automated recommendations, clinical teams can focus on high-value, domain-specific analysis and decision-making, ensuring faster and more informed outcomes. The FDA also has drafted guidelines on the use of AI in clinical research and regulatory submissions.
For organizations looking to implement IDR, the key focus should be on investing in scalable, compliant, and AI-driven solutions, ensuring interoperability with existing systems, and fostering a culture of innovation. Organizations that embrace IDR today will be better positioned to navigate the complexities of modern trials, reduce risk, and enhance patient safety.
About The Author:
Indrani Kakade is a clinical trial data management and analytics consultant with a focus on technology and AI/ML implementation. She is also a senior leader with 30 plus years of experience in clinical research and data operations in global pharma and the outsourcing industry, having held leadership positions at Pfizer Global Research Development, Cognizant Life Sciences, and Sciformix Technologies. Kakade is an early pioneer in outsourcing clinical data management to India, with leadership roles in study setup, data management, statistical programming, and drug safety and pharmacovigilance operations. She is experienced in transition and process management across a wide range of therapeutic areas and trial phases, including Real-World Evidence (RWE), Real-World Data (RWD) and Risk-Based Monitoring (RBM). She also has extensive knowledge in regulatory guidelines (ICH GCP, 21 CFR, etc.) and experience in audits and inspections. She possesses a keen interest in emerging fields and technologies such as digital, social, mobile, data analytics, robotics, and automation.