Busting The Top 7 Data Quality Myths In Clinical Trials
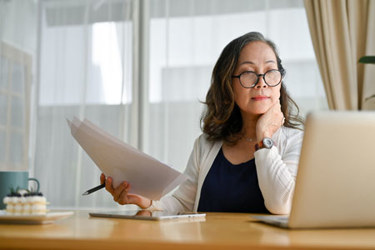
The clinical trial data landscape has transformed due to technology-driven data collection. Diverse data sources are available and expected to grow as trials incorporate new devices and patient participation options. However, the transition and technological evolution hasn’t been easy for teams to adopt.
Notably, diverse sources complicate aggregation. Retrofitting systems or manual methods like spreadsheets are slow and unscalable, and building internal aggregation environments adds complexity. Second, not all data is equal; different sources demand various reviews. Treating all data equally is inefficient and could result in more problems later. Third, but certainly, not least, the rising data volume makes traditional processes less scalable. Not every data point needs a full review, but efficient oversight is vital to the quality of data.
According to an analysis by Medidata, electronic case report form (eCRF) data volume remains steady but has decreased proportionally over time. COVID-19 was also noted to spur remote data collection innovations. Despite pandemic impacts, the industry embraces creativity, learning, and patient-centered approaches in hopes that its continued collaboration will better achieve the integration of data, improve sharing efforts, and break down silos. As aforementioned, however, the data abundance poses operational challenges, raising questions about effective management.
This paper addresses these challenges, separating fact from fiction and dispelling myths about evolving clinical data collection, monitoring, and reporting.
Get unlimited access to:
Enter your credentials below to log in. Not yet a member of Clinical Leader? Subscribe today.